Robotics technology has changed manufacturing a lot. It brings advanced automation solutions that change how factories work. Predictive maintenance is a new way to take care of robots, helping makers avoid problems before they start.
Now, making robots better uses smart algorithms and real-time data. These systems watch important parts of robots, looking for signs of trouble. They track how well robots are doing and find small problems early.
Using data to predict when robots need maintenance helps them last longer. AI looks at how well robots are working and gives tips to fix them before they break down. This way, companies can keep their robots running smoothly for a long time.
Today’s automation uses machine learning to understand robots better. It creates detailed health reports for robots. This smart monitoring finds problems early, cutting down on downtime and making robots work better.
Understanding Predictive Maintenance in Robotics
Predictive maintenance is a game-changer in advanced manufacturing robotics. It changes how we watch over and fix industrial robots. With smart data analysis, robotic engineering services can spot problems before they start.
Today’s robotic systems use advanced monitoring tech. They collect and analyze data in real-time. This turns raw sensor info into useful insights for early maintenance.
Core Components of Predictive Maintenance
Predictive maintenance systems have key parts:
- Sensor networks for constant data gathering
- Advanced machine learning algorithms
- Comprehensive data analysis platforms
- Tools for visualizing and reporting data
Real-time Data Collection Strategies
Manufacturers use many sensors to get detailed performance data. These sensors watch important signs of mechanical trouble.
Sensor Type | Measured Parameter | Significance |
---|---|---|
Vibration Sensors | Mechanical Oscillation | Detects Wear and Misalignment |
Temperature Sensors | Thermal Variations | Identifies Possible Overheating |
Acoustic Sensors | Sound Emissions | Shows Mechanical Problems |
Health Index Monitoring for Robot Joints
Robotic engineering services use health index calculations to check robot joints. These metrics mix different data to give a full picture of performance. This helps focus maintenance efforts.
By using advanced predictive maintenance, makers can cut down on unplanned stops. They also improve how well their robotic systems work.
Industrial Robotics Development and Maintenance Algorithms
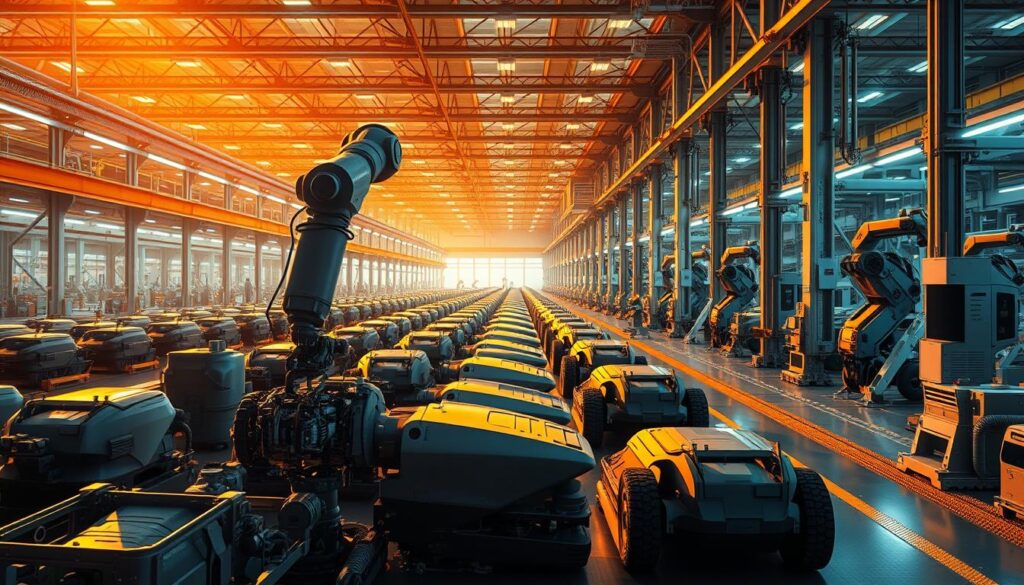
Industrial automation has changed how manufacturers handle robot maintenance and efficiency. Today’s robotic programming includes advanced diagnostic tools. These tools change old maintenance methods.
At the heart of these changes are smart self-checking systems. Robots can spot problems before they get worse. They use complex algorithms to analyze data as it happens. This way, makers can:
- Find equipment failures early
- Reduce unexpected stops
- Plan maintenance better
- Make robots last longer
Machine learning algorithms are key in these systems. They learn from past data to predict issues with high accuracy.
Robot programming now includes smart health checks. It looks at important signs like:
- Vibration patterns
- Temperature changes
- Electric current use
- Mechanical stress signs
These new strategies in industrial automation are a big step up for robot care. They turn old, reactive ways into new, data-based methods. This boosts equipment reliability and performance.
Machine Learning Approaches in Robot Maintenance
Machine learning has changed how we design and build robots. It brings new ways to keep industrial robots running smoothly. These smart methods help monitor, maintain, and improve robot performance.
Robotics is getting better with advanced data analysis. This leads to better maintenance before problems start. Modern machine learning gives deep insights into robot health and wear.
Gaussian Process Regression for Wear Prediction
Gaussian Process Regression is a top method for predicting robot wear. It looks at complex data to:
- Spot early signs of wear
- Guess how long parts will last
- Reduce sudden breakdowns
Hybrid Algorithm Implementation
Using many machine learning methods makes maintenance smarter. Hybrid algorithms mix strengths to improve accuracy and trustworthiness.
Algorithm Type | Predictive Capability | Maintenance Impact |
---|---|---|
Gaussian Regression | High precision wear estimation | Proactive component replacement |
Neural Networks | Complex pattern recognition | Early failure detection |
Ensemble Methods | Comprehensive data analysis | Holistic system health monitoring |
Time Series Analysis and Forecasting
Time series analysis looks at past robot data to predict future performance. Predictive modeling lets engineers plan for maintenance before it’s needed.
These advanced machine learning methods show how robotics innovation changes maintenance. They make robots smarter, more reliable, and more efficient.
Impact of Torque Analysis on Robot Longevity
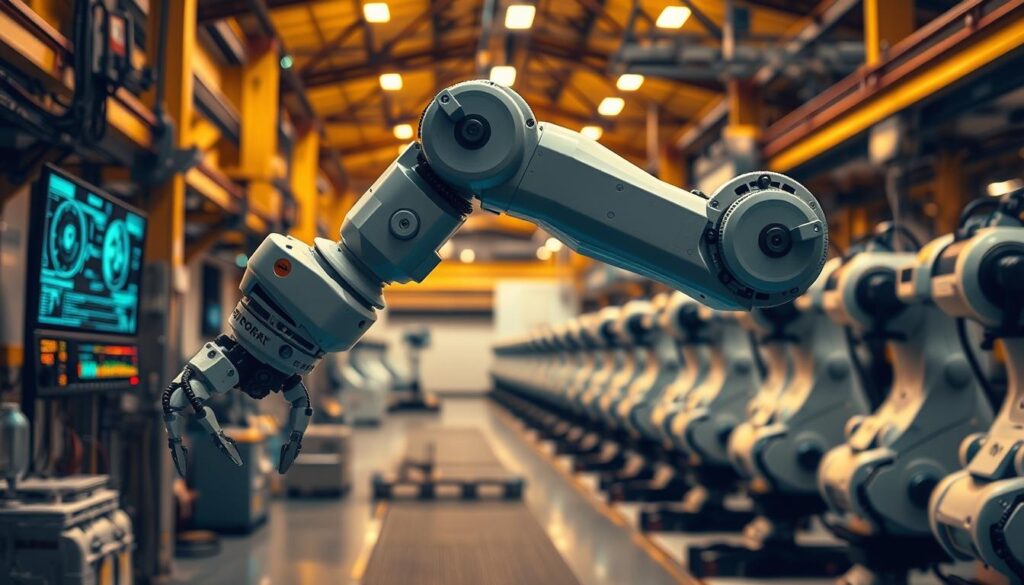
Torque analysis is key in making industrial robots better. It helps makers see how well robots work and when they might need repairs. By watching how much torque each joint uses, engineers can spot problems early and avoid sudden breakdowns.
Understanding stress and strain in robots is at the heart of good automation. Torque tells us a lot about a robot’s health. It shows:
- Small changes in how joints work
- When parts might start to wear down
- When it’s time for maintenance
For example, a company that makes injection molds uses torque analysis well. They use advanced monitoring to cut down on downtime. This lets their team work on making things better and new.
What does torque analysis offer? It helps robots last longer, cuts down on surprises, and makes maintenance better. It also makes production more efficient.
- Longer robot life
- Fewer unexpected failures
- Better maintenance planning
- More efficient production
Now, thanks to new sensors and AI, we can watch robots in real time. This changes how we fix things, making it more about being ready than reacting. It makes robots work better and longer.
Benefits of AI-Powered Maintenance Solutions
Advanced manufacturing robotics have changed industrial maintenance with smart AI solutions. Now, robotic engineering services use the latest tech to improve how manufacturers keep equipment running well.
AI-driven maintenance brings big benefits to industrial work:
- Reduces unplanned machine downtime by up to 50%
- Minimizes unexpected repair costs
- Extends robotic system integration lifecycle
- Improves overall production efficiency
Reduction in Unplanned Downtime
Predictive maintenance algorithms help manufacturers spot equipment problems early. They look at real-time data to predict issues, stopping costly downtime before it starts.
Cost Optimization Through Predictive Repairs
Using AI in advanced manufacturing robotics helps switch to proactive maintenance. Predictive repairs can cut maintenance costs by up to 25%. This lets companies use their resources better.
Enhanced Production Efficiency
AI-powered robotic engineering services keep an eye on key equipment parameters. These smart systems make sure robots work at their best. This boosts productivity and keeps performance high.
Conclusion
Predictive maintenance algorithms are a big step forward in robotics technology. They change how we watch over and fix industrial robots. Now, thanks to advanced data and learning, we can spot problems before they start.
This new way of keeping robots in top shape is thanks to AI. It lets companies track how well their robots are doing very closely. With sensors and smart algorithms, we can catch small issues early, long before we used to.
Robotics is getting smarter and smarter. Companies that use predictive maintenance are saving a lot of money. They get their robots to last longer, fix them cheaper, and work better.
The future of predictive maintenance in robotics looks very bright. As tech gets better, we’ll see even smarter ways to keep robots running smoothly. This will change how businesses handle their robots for the better.